In this article, I analyze a broad range of leading indicators—economic or financial data series that change in advance of the rest of the economy—to see which ones have done better at signaling past U.S. recessions.1 I also use these leading indicators to form a new index that outperforms existing leading indexes and the Treasury yield curve at signaling historical downturns.2
Economists follow many economic and financial data series to gauge the current economic climate and prospects for future activity. My focus here is on leading indicators as signals of U.S. recessions according to the National Bureau of Economic Research (NBER). Specifically, I examine how useful various economic and financial indicators have been in “predicting” recessions in the past and summarize what these indicators suggest about the future. I show that indexes that combine several macroeconomic measures have historically done better than other indicators at signaling recessions (and expansions) up to one year in advance. Additionally, I confirm that financial market measures—especially the slope of the Treasury yield curve—have been useful signals of recessions one to two years ahead of time. Based on historical data, I also compute recession prediction thresholds for all the leading indicators I consider. Then, to combine the information conveyed by these indicators, I compute a new index that shows the share of leading indicators predicting a recession at any given time. This simple index significantly outperforms existing measures at signaling a recession six to nine months in advance.
How to evaluate leading indicators
In this analysis, I assess several leading indicators to find out which ones have been better at predicting recessions in the past based on their historical classification ability of data aligned with future realizations of recessions and expansions. Specifically, I evaluate a list of leading indicators from a variety of sources that are tracked by the Conference Board. These indicators include data on employment, manufacturing activity, housing, consumer expectations, and the return on the stock market. However, I exchange the Conference Board’s measure of differences in Treasury securities’ interest rates across maturities (or the slope of the yield curve) for the more commonly used difference between the ten-year yield and three-month yield (the long-term spread)3 and another version of the yield curve designed to capture expectations of monetary policy (the near-term forward spread).4 I also exchange the Conference Board’s measure of credit conditions for the Chicago Fed’s National Financial Conditions Index (NFCI) and its nonfinancial leverage subindex.5 In addition to the indicators on this list, I analyze the Conference Board Leading Economic Index for the U.S. (the average across its list of indicators); a new leading index two collaborators and I recently produced from a panel of 500 monthly time series and quarterly U.S. real gross domestic product growth called the Brave-Butters-Kelley (BBK) Leading Index;6 the University of Michigan’s Index of Consumer Expectations; the value of debit balances in broker-dealers’ securities margin accounts; and the Standard & Poor’s (S&P) Goldman Sachs Commodity Index (GSCI).7 Throughout this analysis, the 17 indicators I consider have been normalized so that negative values indicate a deterioration in economic activity.8
Ultimately, I want to be able to compare a given observation for any of these indicators with its historical values and then say whether a recession is coming or not. This implies that I am looking for a threshold that the indicator has been always below when signaling a recession (or always above when signaling an expansion). Inevitably, these predictions will be imperfect, and there will be times during a recession when an indicator is greater than the chosen threshold (and times during an expansion when it is less than the threshold). The total fraction of periods an indicator correctly classifies according to a chosen threshold is called its accuracy. Unfortunately, accuracy is a flawed measure for the purpose of recession prediction because correctly classifying a recession is treated the same as correctly classifying an expansion. In the extreme case, a prediction that a recession never occurs is 88% accurate because recessions have only occurred in 12% of all months since 1971. Obviously, it would be preferable to have a predictor that provides a meaningful signal about coming recessions, even though it may be less than 88% accurate.
A better criterion by which one can evaluate these indicators is a statistic known as the area under the receiver operating characteristic (ROC) curve, or AUC value.9 An AUC value measures the classification ability of an indicator based on a pair of data points. Assume we were given two observations of an indicator and were told that one of them is associated with a recession and the other with an expansion. The AUC value is the probability that the lower observation is associated with a recession. As with any probability, AUC values range from zero to one—a value of one means an indicator perfectly classifies a random pair of observations.10 If an indicator is unrelated to coming recessions, there is still a 50-50 chance it correctly predicts a recession, resulting in an AUC value of 0.5.
Because an AUC value is related to random pairs of observations, it is unaffected by the imbalance in the number of recessionary versus expansionary periods observed. Additionally, the AUC value can be computed without first choosing a threshold (unlike accuracy) so that it provides a more robust measure of how much information an indicator conveys about coming economic conditions.
1. AUC values of leading indicators
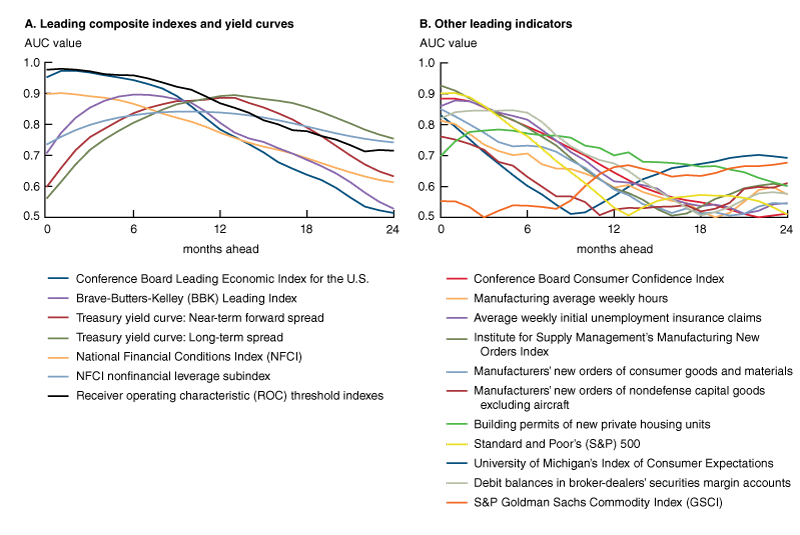
Sources: Author’s calculations based on the data from Haver Analytics and the Board of Governors of the Federal Reserve System.
To evaluate each indicator’s AUC value, I shift the indicators’ observations to align with whether or not a recession occurred a given number of months in the future up to two years ahead. The results are presented as the colored lines in figure 1—with the composite indexes and Treasury yield curve measures (i.e., those with generally higher AUC values) in panel A and the other measures in panel B. Based on these results, I conclude the following:
- Up to nine months in advance, the Conference Board Leading Economic Index for the U.S. does the best at signaling coming recessions and expansions. Based on a statistical test,11 I reject the hypothesis that other indicators are equally as good at predicting a recession one to six months in advance. At seven to nine months ahead, the Conference Board’s leading index remains the best predictor, but I cannot reject a hypothesis that three other indicators are equally as good (the BBK Leading Index and the two yield curve measures). The Conference Board’s leading index is highly accurate in the near term, achieving an AUC value of 0.97 one to three months ahead.
- Far in advance of a recession or expansion, the long-term Treasury yield spread (i.e., ten-year minus three-month Treasury yields) is the best predictor. I can reject the hypothesis that other indicators are equally as good at a horizon of 16 to 20 months ahead. At 14 to 15 and 21 to 24 months ahead, the long-term yield curve slope remains the best predictor, but I cannot reject a hypothesis that at least one of three other indicators are equally as good (the NFCI’s nonfinancial leverage subindex, the S&P GSCI, and the University of Michigan’s Index of Consumer Expectations). Because of the additional uncertainty arising from predicting at longer horizons, the AUC values are lower than those at short horizons: The long-term yield spread achieves an AUC value of 0.89 at 14 months ahead that gradually declines to an AUC value of 0.75 at 24 months ahead.
- At ten to 13 months ahead, several leading indicators produce similar AUC values. The Conference Board Leading Economic Index for the U.S., the BBK Leading Index, the two yield curve slopes, and the NFCI’s nonfinancial leverage subindex all have AUC values between 0.84 and 0.89 at these horizons. Statistical tests are inconclusive as to which one performs the best at these horizons, implying that each should be considered when predicting recessions in the medium term.
- As seen in figure 1, the Conference Board’s leading index and the BBK Leading Index in panel A generally do better at predicting recessions than the macroeconomic indicators featured in panel B. The macroeconomic indicators in panel B perform very poorly at longer horizons, to the point that I cannot reject a hypothesis for many of them that they are equivalent to random noise more than one year ahead. These leading indexes’ AUC values also approach 0.5 at long horizons, but take longer to do so than the macroeconomic indicators'. These observations indicate that the indexes are performing as desired: By minimizing the noise in their component indicators, they provide a clearer signal of future economic activity.
Recession prediction thresholds
While the AUC value is informative about a leading indicator’s general historical classification ability, this measure doesn’t say anything about the threshold that should be used for predicting a recession. The earlier issue I pointed out with accuracy indicates that an alternative approach is needed. To determine this alternative, it is helpful to realize that the choice of the threshold for each indicator separately affects two things: 1) the true positive rate, or how many months ultimately associated with a recession it classifies correctly, and 2) the false positive rate, or how many months ultimately associated with an expansion it fails to classify correctly.
For any threshold I might choose, my goals for these two metrics are in competition with each other. I want to predict as many recessions as possible (achieving a high true positive rate), but I also want as few instances as possible where the indicators “cry wolf” (avoiding a high false positive rate). If I wanted to make sure an indicator was predicting every recession possible, I would choose a high threshold to create a sensitive predictor that gives a high true positive rate at the expense of producing many false recession predictions. Conversely, if I wanted to make sure that a recession was coming when an indicator predicted one, I would choose a low threshold so that only the lowest values of the indicator predict a recession; while this approach would fail to predict some recessions, I would have more confidence of a coming recession when one was predicted.
To resolve this conflict, consider the case of an indicator that provides no information about a coming recession. Whatever threshold is chosen, it simply changes the fraction of the time a recession is predicted. Assume that this random guess predicts a recession 20% of the time. When the results are known if a recession occurred or not, this guess would correctly predict 20% of recessions. However, this guess would also incorrectly predict a recession when an expansion occurred 20% of the time. For such an indicator, this implies that the true positive rate and the false positive rate will always be the same. The more informative an indicator is for a given threshold, the more it will diverge from this relationship. Selecting the threshold that maximizes the difference between true positive and false positive rates provides the most information possible about past recessions for a given indicator.12
Let me go over an example of what this threshold criterion implies for an individual indicator: This “maximum information” threshold for the long-term Treasury yield spread (i.e., ten-year minus three-month Treasury yields) at 12 months ahead is somewhat higher than the traditionally cited value of zero. The zero threshold (otherwise known as a yield curve inversion13) correctly classifies only 57% of recession months and incorrectly classifies 5% of expansion months. The maximum information threshold varies somewhat over the horizons considered, but is constant at 0.94 over the range of eight to 15 months ahead. According to this threshold, the long-term spread one year ahead correctly classifies 88% of recession months, but also incorrectly classifies 19% of months during an expansion.
The choice between these thresholds depends on the context. For those looking to be more confident that a recession is coming when one is predicted, the lower false positive rate of the zero threshold is attractive. The maximum information approach instead focuses on the trade-off between true positive and false positive rates. By raising the threshold, the maximum information approach increases both rates, but more so for the true positive rate than the false positive rate, thus better distinguishing past recessions from expansions.14
A summary index
The maximum information threshold criterion can be applied to each of the indicators at each horizon from zero to 24 months ahead to find an optimal recession prediction threshold. To summarize all of the indicators under consideration as simply as possible, I calculate the fraction of the 17 indicators that are below their optimal threshold and that predict a recession. Effectively, this is a new method of constructing a leading index to predict coming recessions. Notably, this “ROC threshold index” is not an estimated probability of a recession—only the fraction of the indicators considered that have crossed their recession prediction thresholds. To evaluate the 25 ROC threshold indexes, I computed the AUC values for each of them at the corresponding horizon, with the results plotted as the black line in panel A of figure 1.
At horizons of up to 11 months ahead, the ROC threshold indexes are better predictors of coming recessions than any of the series considered.15 Using the same statistical test from before, I can reject a hypothesis that any indicator considered here is as good as these indexes at a horizon of six to nine months. At longer horizons, the predictive ability of the ROC threshold indexes falls below that of the yield curve measures, but remains somewhat informative. Intuitively, the performance of the ROC threshold indexes drops as the predictive ability of the leading indicators used to construct them declines. Because only a few indicators are meaningfully predictive more than a year ahead, the ROC threshold indexes’ ability to discriminate between recessions and expansions deteriorates the further in advance the prediction is made.
The furthest ahead the ROC threshold indexes significantly outperform all other indicators is nine months in advance. Figure 2 plots the times series of the ROC threshold index at this horizon, with the series shifted nine months ahead so that the most recent observation of the data from August 2019 are plotted in May 2020. Determining the appropriate threshold to measure this index against is nontrivial because the objective is not necessarily to extract as much information as possible (as it was with the individual indicators to construct the index). Applying the maximum information approach produces a threshold of 50%. Based on the 50% threshold, this index correctly predicted a recession in 83% of recession months, but incorrectly predicted a recession in 15% of expansion months. Alternatively, a commonly used, more conservative criterion16 produces a threshold of 80%. The 80% threshold has a true positive rate of 26%, but a false positive rate of only 3%. As before, the choice between these thresholds depends on what the prediction will be used for. If one is willing to tolerate a higher likelihood of misclassifying an expansion, the 50% threshold is better; if it is instead more important to be highly confident that a predicted recession is truly coming, the 80% threshold is better. Because both thresholds are potentially useful, they are both plotted in figure 2.
2. ROC threshold index at nine months ahead
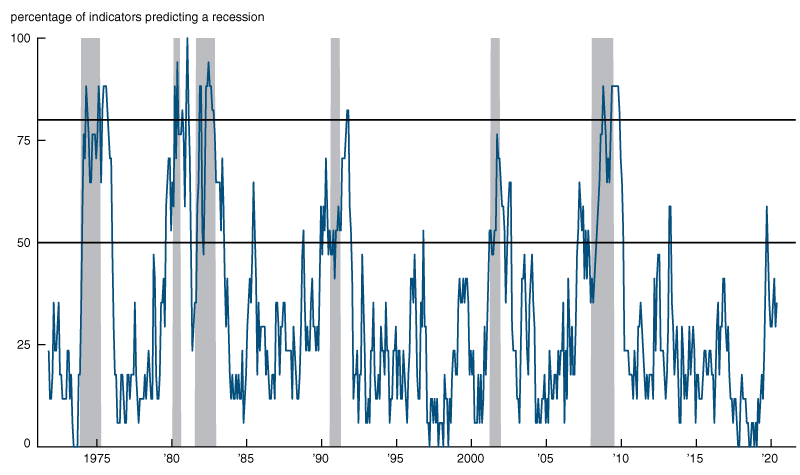
Sources: Author’s calculations based on the data from Haver Analytics and the Board of Governors of the Federal Reserve System.
While the ROC threshold index for nine months ahead rose above 50% based on data observed in December 2018 (plotted in September 2019 in figure 2), it has remained near, but below, the 50% threshold for all data observed since then. This index has remained substantially below the 80% threshold since near the end of the previous recession. Given that this measure is somewhat volatile, these modestly higher recent readings merit some attention, but it remains below the 50% threshold almost always associated with a historical recession.
To be sure, this entire analysis is predicated on the assumption that the data are known with certainty when they are observed. This is clearly not the case, as data are released with a lag and often revised months afterward. A real-time analysis of this approach is necessary to more deeply understand our ability to predict recessions before they occur.
Conclusion
The results of this article show that at horizons roughly one year ahead and longer, the long-term Treasury yield spread has historically been the most accurate available “predictor” of recessions. That said, leading indexes have been better than individual leading indicators or financial data at signaling recessions in the near term. The ROC threshold indexes constructed here have also performed well as recession predictors in the near term because they are also effectively leading indexes that combine the information in the inputs to provide a more accurate measurement of coming economic activity.
Notes
1 I thank Scott Brave for many useful discussions on this material.
2 A yield curve is the line plotting the yields or interest rates of assets of the same credit quality (e.g., high-quality Treasury securities, backed by the U.S. government), but with differing maturity dates at a certain point in time (e.g., short-term Treasury bills versus longer-term Treasury notes and bonds).
3 Arturo Estrella and Mary R. Trubin, 2006, “The yield curve as a leading indicator: Some practical issues,” Current Issues in Economics and Finance, Federal Reserve Bank of New York, Vol. 12, No. 5, July/August, available online.
4 Eric C. Engstrom and Steven A. Sharpe, 2019, “The near-term forward yield spread as a leading indicator: A less distorted mirror,” Finance and Economics Discussion Series, Board of Governors of the Federal Reserve System, No. 2018-055, revised February 2019. Crossref
6 Scott A. Brave, Ross Cole, and David Kelley, 2019, “A ‘big data’ view of the U.S. economy: Introducing the Brave-Butters-Kelley Indexes,” Chicago Fed Letter, Federal Reserve Bank of Chicago, No. 422. Crossref
7 For a more extensive survey of leading indicators, see Weiling Liu and Emanuel Moench, 2016, “What predicts US recessions?,” International Journal of Forecasting, Vol. 32, No. 4, October–December, pp. 1138–1150. Crossref
8 All the indicators I consider are expressed in three-month percent changes with the following exceptions: Conference Board Consumer Confidence Index (untransformed), University of Michigan’s Index of Consumer Expectations (untransformed), Institute for Supply Management’s (ISM) Manufacturing New Orders Index (untransformed), BBK Leading Index (untransformed), the two yield curve measures (untransformed), the NFCI and the NFCI nonfinancial leverage subindex (untransformed), and the Standard and Poor’s (S&P) 500 (12-month percent change).
9 The name area under the ROC curve comes from how the statistic is computed by forming a curve relating the false positive rate of an indicator to its true positive rate at various thresholds and then computing the area under this curve.
10 The normalization used here that lower values of an indicator point to a deterioration in economic activity means AUC values will always be greater than or equal to 0.5.
11 Elizabeth R. DeLong, David M. DeLong, and Daniel L. Clarke-Pearson, 1988, “Comparing the areas under two or more correlated receiver operating characteristic curves: A nonparametric approach,” Biometrics, Vol. 44, No. 3, September, pp. 837–845. Crossref
12 This approach has also been called the “science of the method,” in contrast to the “utility of the method” that explicitly measures the value of each outcome. For details on both approaches, see Stuart G. Baker and Barnett S. Kramer, 2007, “Peirce, Youden, and receiver operating characteristic curves,” American Statistician, Vol. 61, No. 4, November, pp. 343–346. Crossref
13 An inversion in the Treasury yield curve has occurred when interest rates on long-term Treasury securities have become lower than those on short-term Treasury securities.
14 In contrast, the “utility of the method” approach (see note 12) focuses on the trade-off between the counts of true positive and false positive events. The connection between the two is illustrated by considering the utility weights on prediction outcomes used to construct the thresholds. For these utility weights, three values need to be assigned: 1) the relative utility of correctly predicting an expansion when one occurs to the disutility of incorrectly predicting a recession; 2) the relative utility of correctly predicting a recession when one occurs to the disutility of incorrectly predicting an expansion; and 3) the relative utility for outcomes associated with periods of expansions versus recessions. The “utility of the method” approach typically assigns equal utility weights to each prediction (see Travis J. Berge and Òscar Jordà, 2011, “Evaluating the classification of economic activity into recessions and expansions,” American Economic Journal: Macroeconomics, Vol. 3, No. 2, April, pp. 246–277. Crossref). The maximum information approach (or the “science of the method”) is equivalent to correcting for the fact that recessions are relatively rare by setting the first and second values to be equal and then setting the third value to be the inverse of the ratio of expansions to recessions (therefore putting a utility weight roughly seven times larger on recessions, given that they occur roughly one-seventh as often as expansions).
15 Indexes constructed using the “utility of the method” (see notes 12 and 14) performed poorly by comparison, never achieving a better AUC value than the best leading indicator considered.
16 The more conservative threshold is based on the “utility of the method” as opposed to the “science of the method” that is equivalent to the maximum information approach (see notes 12 and 14).