The Covid-19 pandemic has had an enormous impact on the U.S. economy. Nowhere are the effects more dramatic than in the labor market: In a span of just two months, the unemployment rate increased from 3.5% in February 2020—a low not seen since the late 1960s—to 14.7% in April—a high not seen since the Great Depression—before falling modestly in May and June. How persistent are these effects likely to be? Will the labor market recover quickly once pandemic-related restrictions are fully lifted, or will unemployment remain at elevated levels further into the future?
One important consideration as we assess the persistent impacts of Covid-19 is that the pandemic has also been a reallocation shock: Because the pandemic has had disproportionate effects on different industries, it may lead to a reshuffling of workers across those industries.1 Because inter-industry reallocation is more difficult and time-consuming than within-industry reallocation (due, e.g., to necessary retraining and relocation), the reallocation induced by the pandemic may lead to a higher level of unemployment for a more extended period.
In this Chicago Fed Letter, I calculate a quantitative assessment of this hypothesis. The analysis proceeds in three steps. First, I construct a measure of cross-industry differences—i.e., dispersion—in the response to common shocks using data on industry-level stock market returns. Second, I build a statistical model relating the dispersion measure to the aggregate unemployment rate at various future horizons. In the third step, I apply the model to the actual dispersion realized in the first months of the Covid-19 era to calculate the potential effects of the Covid-induced reallocation on short- and medium-run unemployment.
The results show that by increasing the rate of worker reallocation, the Covid-induced dispersion may have a significant detrimental impact on unemployment in the short and medium term. The effects increase up to a horizon of two years after the initial shock and fade out after about three years. The analysis suggests that at the peak, the reallocation caused by the Covid-19 crisis may contribute about 2 percentage points to the unemployment rate, with the estimates ranging from 0.85 to 4 percentage points. In this article, I provide an overview of the analysis and main results. An accompanying technical appendix provides further details.2
Measuring cross-industry dispersion
I construct a measure of cross-industry dispersion using data on industry-level stock market returns. Stock returns are a natural way to measure shocks that lead to inter-industry reallocation: In principle, movements in stock prices are purely forward-looking measures of expected future performance and shift immediately in response to new information.3 Further, financial market data are a potentially valuable source of evidence on the effects of the pandemic since they are available at a high frequency and in essentially real time.
Figure 1 illustrates the basic idea. The figure plots cumulative stock returns across 49 industries between February 21, 2020 (the all-time high of the S&P 500), and April 30, 2020. Over this period, the return on the aggregate (value-weighted) market (labeled market in the figure) was about –15%. However, that number masks a large dispersion across industries: For example, the hardest-hit industries—aircraft, coal, and fabricated products—saw declines of over 35%, while industries such as pharmaceutical products and retail actually experienced slight increases (precious metals is a clear outlier). The standard deviation of cumulative returns across these industries is over 11%. Clearly, the data suggest that Covid-19 is expected to have very different effects across different sectors of the economy.
1. Industry-level stock returns from February 21, 2020, to April 30, 2020
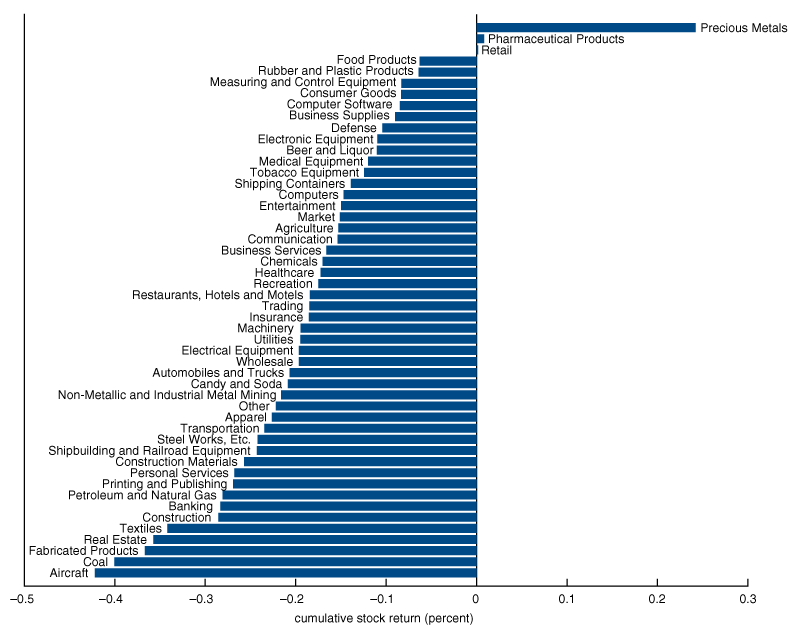
Dispersion and unemployment
I measure the industry-level effects of Covid-19 using data on stock market returns. In principle, cross-industry dispersion in returns is due to two forces: different responses to common shocks, which I call csv_agg (short for “aggregate cross-sectional volatility”), and idiosyncratic shocks that are specific to each industry. In the technical appendix, I outline a method for separately measuring these two components of dispersion. It seems reasonable to assume that relative stock price movements since the onset of Covid-19 (i.e., since February 2020) largely reflect the differential exposures of industries to the single Covid shock and so are captured by csv_agg. Thus, throughout the analysis I use csv_agg as the measure of dispersion. Figure 2 plots the historical series of csv_agg from January 1948 through April 2020. Although by no means the historical high, dispersion clearly jumps in the Covid-19 months—from essentially zero in January 2020 to 0.024 in February, and about 0.04 in both March and April.4
2. Historical series of csv_agg
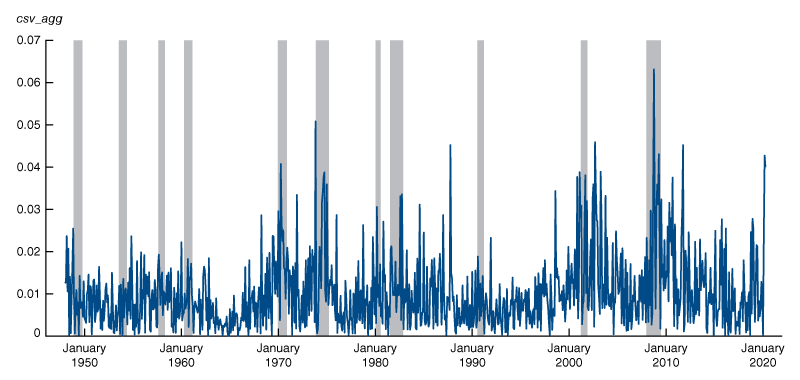
Source: Author’s calculations based on stock return data from Kenneth French’s data library, available online.
Next, I relate cross-industry dispersion to labor market conditions by estimating regressions of the future unemployment rate on the csv_agg measure of cross-sectional dispersion. Specifically, I regress the future unemployment rate at month t + h, h = 3, 6, ..., 45, 48 on the values of csv_agg at a number of lags (specifically, at date t, t − 1 and t − 2), which allows the unemployment rate to depend on the realizations of csv_agg over multiple months.5 Additionally, I control for current levels of the unemployment rate and the aggregate market return. This approach allows for persistence in the unemployment rate and distinguishes the effects of dispersion in returns from the level of the market return. Note that my goal here is not necessarily to formulate the best overall predictor of future unemployment, but rather to simply control for other factors that may be correlated with the dispersion measure in order to obtain an accurate estimate of its effect alone. I report the detailed estimates from these regressions in the technical appendix. The results show that higher dispersion typically signals increases in future unemployment. The estimates tend to be statistically significant at standard confidence levels for between three and about 30 months, with a peak effect at 24 months.
Application to the Covid-19 period
I calculate the implications for unemployment following the Covid-19 pandemic by applying the estimated coefficients to the return dispersion (i.e., csv_agg) realized in the Covid-19 months to date, namely February through April 2020. Figure 3 illustrates the main results. The figure shows the incremental unemployment rate—the additional unemployment stemming from the heightened dispersion realized in the three Covid-19 months—at horizons of three to 48 months. The solid line is the point estimate and the shaded region the 95% confidence interval around that estimate. The figure suggests a significant effect of Covid-induced dispersion on future unemployment, both in a statistical sense and an economic one. The confidence intervals exclude zero up to 33 months (11 quarters), implying that the effects may last approximately three years. The peak impact occurs at 24 months, reaching a maximum of about 2 percentage points of additional unemployment. In sum, the results suggest that inter-industry reallocation (at least as suggested by stock market data) may contribute to an elevated level of unemployment for the next three years, with a peak contribution of about 2 percentage points occurring in two years.
3. Predicted unemployment from Covid-induced reallocation
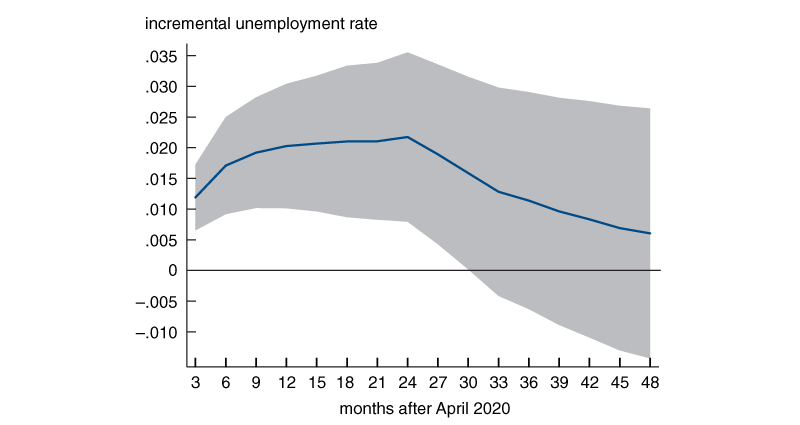
Additional results
The technical appendix reports additional results from a number of alternative scenarios. First, the results in figure 3 may be conservative in attributing a significant portion of dispersion during the Covid-19 era to idiosyncratic industry conditions, rather than to heterogeneity in industry responses to the common Covid-19 event. In the technical appendix, I calculate an alternative estimate where I assume that all dispersion in the February to April 2020 period is due to Covid-19. Because the statistical relationship between dispersion and unemployment is unchanged, the path of the effects (e.g., duration and date of peak) is the same. However, the magnitudes are substantially larger: The peak impact on the unemployment rate reaches as high as about 4 percentage points. Thus, if one is willing to assume that all cross-industry dispersion observed in the Covid-19 months is due to the pandemic (which may not be unrealistic), the potential effects on unemployment could be even more substantial.
Second, although frictions in the process of reallocating workers across industries is a natural channel linking the heterogeneous response to shocks to the level of unemployment, there may be other factors at work as well. For example, measures of cross-sectional dispersion in stock returns are often used as proxies for economic uncertainty—if heightened uncertainty leads firms to reduce hiring and/or changes job search behavior on the part of workers, that may also account for some portion of the link between dispersion and unemployment. Put another way, the analysis above implicitly assigns the reallocation interpretation to explain the entire relationship between dispersion and unemployment, when in reality some of this relationship may not be due to increased cross-industry reallocation. Although precisely disentangling these forces and pinpointing the role of reallocation is challenging, in the technical appendix I present one calculation along these lines.
The procedure is as follows: First, I construct an index of actual employment reallocation across industries. Next, I perform the same regressions as above but add the reallocation index as an additional control variable (at each horizon of the unemployment rate, I include the employment reallocation index over that same horizon). The difference in the effects of csv_agg between the baseline approach and this second estimate can be interpreted as the total effect of dispersion less the part that comes through forces other than reallocation, thus representing a rough measure of the effects due to reallocation alone. Using this result, I can calculate the effects of the Covid-induced dispersion on unemployment due solely to inter-industry reallocation. The results continue to show a significant effect of dispersion-based reallocation on unemployment. The magnitudes are generally slightly under half of the baseline estimates, with the effects increasing up to 24 months—the same peak as in the baseline case—and falling thereafter. At the maximum, the results imply between 0.85 and 1.5 additional percentage points on the unemployment rate.6
Caveats and conclusions
There are some clear caveats to the analysis. First, I have assumed that the historical relationship between dispersion and unemployment will hold following the Covid-19 shock. There are a number of reasons why this may not be the case. In particular, if the Covid-induced downturn is larger but less persistent than other business cycles, return dispersion may not signal a “typical” pattern of reallocation and elevated unemployment. Second, the results are somewhat sensitive to assumptions on dispersion, as well as the statistical model and controls used to evaluate its effects. There are arguments to be made that the full set of results bound the potential effects. One consistent message is that the effects tend to last about three years with a maximum impact at about two years. I have focused on cross-industry dispersion and reallocation, but recent papers have shown that the Covid-19 crisis has also led to disproportionately high dispersion across firms within industries.7 If intra-industry reallocation is also subject to frictions and Covid-19 leads to increased reallocation at the firm level, the impact on the labor market may be larger than calculated here.
In sum, my findings suggest that the inter-industry reallocation of labor caused by the Covid-19 pandemic may have a significant and persistent detrimental effect on the aggregate rate of unemployment. Using up-to-date labor market data to look for direct evidence of increased cross-industry worker flows would be a valuable way to provide additional perspective on the likelihood of this scenario playing out as forecasted. Updating the estimates using incoming financial market data may provide more insight as to the future effects of the pandemic on the labor market. Further, using more-sophisticated econometric techniques/models to more accurately distinguish the effects of dispersion through reallocation as opposed to other channels may be possible.
Notes
1 See, e.g., Jose Maria Barrero, Nicholas Bloom, and Steven J. Davis, 2020, “Covid-19 is also a reallocation shock,” National Bureau of Economic Research, working paper, No. 27137, May, Crossref, for various pieces of empirical evidence.
3 An existing literature has examined the reallocation hypothesis using measures of stock return dispersion, e.g., Prakash Loungani, Mark Rush, and William Tave, 1990, “Stock market dispersion and unemployment,” Journal of Monetary Economics, Vol. 25, No. 3, June, pp. 367–388, Crossref, and S. Lael Brainard and David M. Cutler, 1993, “Sectoral shifts and cyclical unemployment reconsidered,” Quarterly Journal of Economics, Vol. 108, No. 1, February, pp. 219–243, Crossref. Daniel Aaronson, Ellen R. Rissman, and Daniel G. Sullivan, 2004, “Can sectoral reallocation explain the jobless recovery?,” Economic Perspectives, Federal Reserve Bank of Chicago, Vol. 28, Second Quarter, pp. 36–49, available online, provide an excellent overview of various approaches to measuring dispersion in sectoral shocks.
4 The technical appendix provides an analogous figure for the historical series of total cross-industry return dispersion.
5 I have also worked with alternative timing assumptions and obtained similar results.
6 The employment reallocation index is computed using a set of more aggregated industries (14 industries) than the stock market-based measure of dispersion (49) and so may yield an underestimate of the true extent of inter-industry reallocation. In this case, the results in the figure may be interpreted as a lower bound of the impact of Covid-19 on unemployment due to reallocation.
7 See, e.g., Barrero, Bloom, and Davis (2020).